A couple of years after its initial boom, artificial intelligence (AI) still remains a huge buzzword in the fintech industry, as every firm looks at a new way of integrating the tech into its infrastructure to gain a competitive edge. Exploring how they are going about doing this in 2025, The Fintech Times is spotlighting some of the biggest themes in AI this February.
Throughout February, we have discussed extensively how AI is being used to accelerate back-office operations, customer interactions and more. However, AI isn’t a problem-free solution. Hearing from experts across the industry, we delve into some of the biggest challenges of using AI.
Monitoring AI so it doesn’t get outsmarted by fraud
AI is constantly learning and adapting to offer a more personalised solution. However, as James Lichau, financial services co-leader at BPM, the accounting service provider, said, firms must be vigilant and watch over AI services so they don’t get outsmarted by ever-developing fraud tactics.
“While AI presents immense opportunities for the fintech industry, it also raises significant challenges and limitations. The use of AI in fintech has sparked concerns about data privacy and the misuse of sensitive financial information. It necessitates robust safeguards and adherence to data protection regulations.
There is a growing demand for transparent and explainable AI models.
“This is particularly true in the financial sector, where trust and accountability are paramount. Fintech companies must prioritise the development of interpretable AI systems and provide clear rationales for their decisions.
“As cyber threats and fraud tactics evolve, fintech firms must remain vigilant. This means continuously updating and retraining their AI models to stay ahead of malicious actors. It’s vital that they maintain the integrity of their systems. Addressing these challenges is crucial for AI’s responsible and sustainable integration in the fintech landscape.
Identifying fraud tactics using AI

AI is a double-edged blade. While it can be extremely helpful in preventing fraud, in the wrong hands, it can also facilitate it.
Exploring what firms must do, Nick Campbell, chief product officer of payments at Clearent by Xplor Technologies, the SaaS and embedded finance platform, said: “While AI is an incredibly helpful tool for combating fraud, it is also an immensely powerful tool for committing fraud too. A big focus for our security and risk teams in the next year will be ensuring we stay connected to the best practices identified in cyber fraud and maintain the integrity of our payments infrastructure.”
Balancing human oversight and automation

For Swapnil Shinde, CEO at Zeni, an AI bookkeeping software backed by a dedicated finance team, organisations walk a fine line between over-utilising AI that lacks empathetic decisions and relying on humans where errors can be made.
“Among the greatest challenges posed by the use of AI in fintech is the challenge of balance between automation and human oversight. While AI brings greater efficiency and fewer mistakes, human judgment in many areas is still indispensable—especially those requiring subtle financial decisions.
“Another big challenge is related to data security and privacy. AI works through vast reams of data to perform its functions well, and the security of the data and responsible use are essential. The regulatory frameworks around AI in fintech are just evolving, and navigating through this change will be just as important to keep businesses ahead.”
Thoughtful governance and proactive risk management
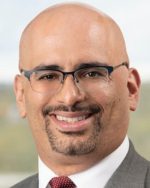
There is a misconception that as AI learns from data, it will be impossible for it to make mistakes. Charles Nerko, team leader for data security litigation in Barclay Damon LLP, the law firm, explains how this is not the case, and why firms need to be proactive in managing the risk in a compliant manner.
“AI brings significant legal challenges to the fintech sector. A top concern is liability for AI errors. AI systems function as ‘black boxes’, making decisions difficult to trace. AI-induced errors, such as biased loan approvals or inaccurate financial information, can lead to lawsuits for discrimination or consumer deception.
“Keeping pace with evolving laws is another challenge. AI regulations are nascent, with a growing, fragmented patchwork of federal, state, and industry-specific rules. Staying ahead of new regulations and following industry best practices are necessary to avoid regulatory scrutiny, litigation, and reputational damage.
“AI contracts compound these risks if poorly structured. Contracts need to address AI-specific risks to avoid leaving organisations vulnerable. Contracts should clearly define performance and confidentiality standards for AI tools as well as delineate responsibilities for when an AI-created problem arises.
“Thoughtful governance and proactive risk management allow AI to be confidently leveraged in a highly regulated environment.”
Importance of complying with regulations

Sharing a similar sentiment, Krishna Venkatraman, chief data officer at Kueski, the buy-now-pay-later (BNPL) firm, added: “One of the biggest challenges the fintech sector will face as AI gains popularity will be the development and implementation of regulations. In 2024, we saw many ‘first-of-its-kind’ AI regulations, such as the EU AI Act and the proposed California AI bills.
“As society becomes increasingly aware of the power of AI models, their rapid proliferation and their widespread use, more guidelines will come into play as an attempt to not only preserve a path for ongoing innovation but to also limit the substantial damage that these technologies can wreak in the hands of malicious or bad actors. In 2025, I believe we’ll see a period of sustained regulatory activity and adaptation as agencies attempt to strike the right balance between enforcing regulations and encouraging innovation.”
Breaking down data silos

AI only works if it is constantly being fed new data and information to work off of. Jason Pedone, chief technology officer at Aspida, the insurance firm, notes that implementing the correct systems to make this a seamless process can often be a hurdle firms trip up on.
“The primary challenge most organisations will need to overcome is breaking down data silos. The ability to continuously feed data to support AI systems is a complex task that most organisations underestimate. Newer organisations have an advantage here, given that they tend to have significantly less tech debt and employ modern tech stacks and data formats.
“Another significant set of challenges is building AI systems that remain adaptable to evolving regulations, developing robust security protocols to protect sensitive financial data, and maintaining the right balance between automation and human oversight. Organisations must navigate data privacy concerns, maintain transparency in AI decision-making processes, and ensure their systems remain unbiased and fair.
“The rapid pace of AI advancement also creates pressure to continuously update and improve systems while managing implementation costs and ROI expectations.”
AI doesn’t instantly mean profitability
For Farooq Khan, VP-senior analyst at Moody’s Ratings, AI is no longer a nice-to-have – it is a necessity. However, simply implementing it doesn’t solve every company problem. AI is just one cog in a much larger machine that will result in profitability, but ensuring the tech works properly can be costly.
“Integrating AI into banking is both technically and financially demanding due to strict regulations, legacy systems, and complex processes. AI systems require high-quality data for accurate decision-making, necessitating data consolidation at scale and cleaning to ensure usability.
“The quality of a bank’s IT infrastructure is a key component in the success of AI adoption, but because fintechs do not have complicated legacy IT infrastructure built up over several decades, tending to be cloud native at start, embedding AI technologies may prove to be less cumbersome that for larger banking institutions.
“Regulatory considerations are another factor, as fintechs would need to navigate complex compliance requirements while leveraging AI technologies. Operational risks would also arise from AI’s extensive data needs, leading to scalability and interoperability challenges, while centralisation risks can create single points of failure, weakening system resilience. Rapid AI advancements also introduce technology risks, as financial firms must continuously invest in infrastructure to prevent obsolescence.
“Moreover, profitability remains a challenge for many fintech firms that tend to lag larger banks in this regard and have taken time to become profitable. This is in large part due to the fierce competition with tech-savvy incumbent banks and many other fintechs for clients, market share, financial and people resources, as well as access to equity. As a result, despite significant investments in AI, fintechs would require a strategic approach to AI integration, balancing innovation with risk mitigation.”